Sesgos en el Pronóstico del Precio en el Par de Divisas EURUSD: Evaluación Algorítmica y Gráfica de los Osciladores Estocástico y MACD
Palabras clave:
Par de divisas EUR/USD, Análisis algorítmico, Osciladores Estocástico y MACD, Pronóstico del precio, Trading de divisas, Inteligencia artificial, Sesgos interpretativos, Toma de decisiones en el tradingSinopsis
En el vasto y multifacético universo de los mercados financieros, el par de divisas EUR/USD emerge como un coloso, un ente que no solo refleja las complejidades económicas de Europa y Estados Unidos, sino que también actúa como un barómetro de la estabilidad y las tendencias financieras globales. Este libro, "Sesgos Técnicos en el Pronóstico del Precio en el Par de Divisas EURUSD: Evaluación Algorítmica de los Osciladores Estocástico y MACD", es una obra dedicada a evaluar la dinámica de este par de divisas, a través de la evaluación algorítmica y gráfica.
La interacción entre el euro y el dólar estadounidense es mucho más que una mera transacción financiera. Representa un complejo entrelazado de políticas económicas, respuestas del mercado y, de manera crucial, las percepciones y reacciones psicológicas de los inversores a nivel mundial. En este contexto, la tarea de predecir los movimientos de precios en este par de divisas se convierte en un desafío formidable, plagado de incertidumbres y sesgos interpretativos.
Este libro surge de la necesidad de comprender mejor estos desafíos, con el fin de orientar a inversores principiantes, estudiantes y académicos. En la presente investigación, se examinan y evidencian los errores comunes que pueden producirse en el pronóstico del precio basado en la información brindada por un solo indicador, y cómo estos se manifiestan en el gráfico. Como parte del estudio, se utilizarán para este fin los osciladores técnicos tradicionales y muy conocidos en el campo financiero, tales como el Estocástico y el MACD, siendo evaluados con un enfoque particular en el par EUR/USD. La metodología de backtesting, un pilar en el modelado financiero se emplea para revelar la verdadera naturaleza de las señales de estos indicadores, ofreciendo una visión más clara de su fiabilidad y eficacia, al igual que el análisis gráfico, que se enfoca en la interpretación del movimiento del precio a partir de su observación.
El propósito de esta obra es doble. Primero, busca proporcionar a los operadores e inversores una comprensión más profunda y matizada de las herramientas que utilizan diariamente. Al identificar y comprender los sesgos en la interpretación de estos osciladores teniéndolos como única fuente de información, se puede mejorar significativamente la toma de decisiones en el trading, reduciendo el riesgo y maximizando las oportunidades de inversión rentable. Segundo, este libro invita a repensar y reevaluar las metodologías tradicionales que se han convertido en estándares en el mundo del comercio online de divisas.
La estructura del libro está cuidadosamente diseñada para guiar al lector a través de un viaje de descubrimiento y aprendizaje:
Capítulo 1, se aborda a manera general conceptos respectos al análisis algorítmico y backtesting, la euforia y el pánico en el mercado de divisas, lo que implica el análisis gráfico o chartismo, el análisis técnico y los osciladores en este contexto.
Capítulo 2, se centra en el Oscilador Estocástico, evaluando algorítmicamente las señales de compra y venta en el par EUR/USD e identificando los posibles errores en la toma de decisiones observados en el gráfico a partir de su única y estricta interpretación. Este capítulo desglosa la metodología y presenta los resultados de la evaluación.
Capítulo 3, examina el Oscilador MACD, evaluando los cambios de tendencia en el par EUR/USD e identificando los posibles errores en la toma de decisiones observados en el gráfico a partir de su única y estricta interpretación. Aquí, se analiza la eficacia del MACD en la identificación de tendencias y se discuten los resultados obtenidos.
Capítulo 4, destaca la relevancia del análisis técnico en la interpretación de los mercados financieros modernos y la persistente importancia de los gráficos de precios para comprender las tendencias del mercado. Aborda los desafíos asociados con la fiabilidad de los backtests en el trading algorítmico y examina cómo el crecimiento y las innovaciones en este campo están siendo impulsados por tecnologías avanzadas como la inteligencia artificial y el aprendizaje automático. Además, enfatiza el rol de los algoritmos en la mitigación de sesgos en el análisis financiero, especialmente en estudios retrospectivos como el del par EUR/USD.
Este libro no es solo una exploración técnica; es una invitación a embarcarse en un viaje de conocimiento y reflexión crítica. Es una herramienta indispensable para aquellos que buscan navegar con éxito en el complejo y a menudo turbulento mundo de las finanzas y los mercados de divisas. Con un análisis riguroso y una perspectiva crítica, este libro promete enriquecer su comprensión y mejorar su enfoque en el trading de divisas.
Bienvenidos a una obra que no solo ilumina el camino en el comercio online de divisas, sino que también desafía y expande nuestra comprensión de los mercados financieros globales.
Capítulos
-
CAPÍTULO 1. EL PRONÓSTICO DEL PRECIO: UNA PERSPECTIVA TÉCNICA Y PSICOLÓGICA EN EL MERCADO DE DIVISAS
-
CAPÍTULO 2. EVALUACIÓN ALGORÍTMICA Y VISUALIZACIÓN GRÁFICA DE SESGOS EN LA INTERPRETACIÓN DEL OSCILADOR ESTOCÁSTICO PARA EL PRONÓSTICO DE PRECIOS DEL EUR/USD
-
CAPÍTULO 3. EVALUACIÓN ALGORÍTMICA Y VISUALIZACIÓN GRÁFICA DE SESGOS EN LA INTERPRETACIÓN DEL OSCILADOR MACD PARA EL PRONÓSTICO DE PRECIOS DEL EUR/USD
-
CAPÍTULO 4. EVALUACIÓN HISTÓRICA DE LA ESTRATEGIA Y PRONÓSTICO DEL PRECIO UN PRODUCTO FINANCIERO: DEL TRADING MANUAL AL ALGORÍTMICO
-
REFERENCIAS BIBLIOGRÁFICAS Y ANEXOS
Descargas
Citas
Ahmed, S., Hassan, S., Aljohani, N., & Nawaz, R. (2020). FLF-LSTM: a novel prediction system using Forex Loss Function. Applied Soft Computing, 97, 106780. doi:https://doi.org/10.1016/j.asoc.2020.106780
Aji, S., Ternanda, E., Bagus, R., & Bagus, Z. (2022). The Marketing Strategy and Benefits Analysis of Papringan Market in Indonesia: A Narrative Review of Literature. Urecol Journal.Part H: Social, Art, and Humanities, 2(2), 44-48. doi:https://doi.org/10.53017/ujsah.169
Almeida-Palacios, D. A., Calderero-Villagómez, E. A., & Reyes-Vélez, P. E. (2019). Mercado de divisas trading forex bolsa de valores. Dominio de las Ciencias, 5(3), 528–548. doi:https://doi.org/10.23857/dc.v5i3.951
Appel, G. (2005). Technical analysis: power tools for active investors. Upper Saddle River: FT Press.
Atiah, F. D., & Helbig, M. (2019). Effects of Decision Models on Dynamic Multi-objective Optimization Algorithms for Financial Markets. EEE Congress on Evolutionary Computation (CEC) (págs. 762-770). Wellington, New Zealand: Institute of Electrical and Electronics Engineers - IEEE. doi:doi: 10.1109/CEC.2019.8790275
Ayitey Junior, M., AAppiahene, P., & Appiah, O. (2022). Forex market forecasting with two-layer stacked Long Short-Term Memory neural network (LSTM) and correlation analysis. Journal of Electrical Systems and Inf Technol, 9(14). doi:https://doi.org/10.1186/s43067-022-00054-1
Ayitey, J. M., Appiahene, P., & Appiah, O. e. (2023). Forex market forecasting using machine learning: Systematic Literature Review and meta-analysis. J Big Data, 10(9). doi:https://doi.org/10.1186/s40537-022-00676-2
Bank for International Settlements. (24 de Septiembre de 2019). bis. Obtenido de https://www.bis.org/statistics/rpfx19_fx.pdf
Batten, J., Loncarski, I., & Szilagyi, P. (2021). Strategic insider trading in foreign exchange markets. Journal of Corporate Finance, 69. doi:https://doi.org/10.1016/j.jcorpfin.2020.101818
Bayguzina, L., Galimova, G., & Safina, Z. (2020). Influence of the Financial Market in the Conditions of Economic Globalization. International Scientific Conference "Far East Con" (ISCFEC 2020). 128, págs. 453-458. Atlantis Press SARL. doi:10.2991/aebmr.k.200312.065
Bernards, N. (2023). Capturing Finance: Arbitrage and Social Domination. Contemporary Sociology, 52(2), 149-150. doi:https://doi.org/10.1177/00943061231155321p
Cersosisimo, F. (2013). High-Frequency Trading and Broader Implications for Strategic Asset Allocation. SSRN. Obtenido de https://ssrn.com/abstract=2216950
Chen, J. (18 de 08 de 2021). Investopedia. Obtenido de https://www.investopedia.com/terms/b/backtesting.asp
Chen, J. (04 de 03 de 2021). Investopedia. Obtenido de https://www.investopedia.com/terms/o/oscillator.asp
Chen, J. (29 de 08 de 2021). Investopedia. Obtenido de https://www.investopedia.com/terms/t/technicalindicator.asp
Cheung, Y., & Chinn, M. (2001). Currency traders and exchange rate dynamics: a survey of the US market. Journal of International Money and Finance, 20(4), 439-471. doi:doi:https://doi.org/10.1016/S0261-5606(01)00002-X
Cootner, P. (1967). The random character of stock market prices. Cambridge (MA): MIT Press.
Dasanayake, D. M., Dilshan, H. Y., Rathnaweera, H. D., Ahangama, S., & Perera, I. (2023). Novel Approach for Deep Learning-Powered Forecasting of Market Bottoms in Cryptocurrency and Stock Trading. IEEE International Conference on Big Data (BigData) (págs. 1481-1486). Sorrento, Italy: IEEE Xplore. doi:10.1109/BigData59044.2023.10386273
Deep Learning for Algorithmic Trading: Enhancing MACD Strategy. (2020). ICCAI '20: Proceedings of the 2020 6th International Conference on Computing and Artificial Intelligence (págs. 51–57). New York, NY, USA.: Association for Computing Machinery. doi:https://doi.org/10.1145/3404555.3404604
Deloitte. (2021). Ciencia de datos y algoritmos aplicados a la gestión financiera: oportunidades y riesgos. Obtenido de https://www.eleconomista.com.mx/empresas/Ciencia-de-Datos-y-Algoritmos-en-la-Gestion-Financiera-20210514-0049.html
Dierks, L., & Tiggelbeck, S. (2021). Finanzas emocionales: el impacto de las emociones en las decisiones de inversión. ournal of New Finance, 2(2). doi:DOI: 10.46671/2521-2486.1019
do Prado, H., Ferneda, E., Morais, L., Luiz, A., & Matsura, E. (2013). On the Effectiveness of Candlestick Chart Analysis for the Brazilian Stock Market. Procedia Computer Science, 11, 1136-1145. doi:https://doi.org/10.1016/j.procs.2013.09.200
Dolan, B. (19 de 12 de 2023). Investopedia. Obtenido de https://www.investopedia.com/terms/m/macd.asp
Downey, L. (31 de 07 de 2023). Investopedia. Obtenido de https://www.investopedia.com/terms/a/algorithm.asp
FasterCapital. (12 de 12 de 2023). fastercapital. Obtenido de https://fastercapital.com/es/contenido/Superar-el-sesgo-de-anticipacion--estrategias-para-una-toma-de-decisiones-precisa.html
Galeshchuk, S., & Mukherjee, S. (2017). Deep learning for predictions in emerging currency markets. ICAART 2017 - Proceedings of the 9th International Conference on Agents and Artificial Intelligence, (págs. 681–686).
Gallegos-Erazo, F. (2022). Análisis Chartista de los Futuros E-Mini Nasdaq-100 Durante el Colapso del Mercado de Valores en el 2020. Universidad y Sociedad, 452-461. Obtenido de https://rus.ucf.edu.cu/index.php/rus/article/view/2649
Gallegos-Erazo, F. (2022). Technical Indicator for a Better Intraday Understanding of Uptrends or Downtrends in the Financial Markets using Volume Transactions as a Trigger. ICAIW 2022: Workshops at the 5th International Conference on Applied Informatics 2022, (págs. 182-194). Arequipa, Perú. Obtenido de https://ceur-ws.org/Vol-3282/icaiw_aiesd_11.pdf
Gallegos-Erazo, F. (2024). Bullish Price Patterns in the NASDAQ-100 Stock Index Evaluated Through Genetic Algorithm. SN Computer Science, 53(5). doi:https://doi.org/10.1007/s42979-023-02430-8
Gonz, C., & Herman, M. (2018). Foreign exchange forecasting via machine learning. Obtenido de https://cs229.stanford.edu/proj2018/report/76.pdf
Gulzar, T., & Ali, N. (2023). La influencia de los sesgos de comportamiento en las decisiones de inversión; Papel moderador de la estabilidad emocional. Revista de Desarrollo y Ciencias Sociales, 4(2), 692–708. doi:https://doi.org/10.47205/jdss.2023(4-II)61
Hayes, A. (29 de 07 de 2023). Investopedia. Obtenido de https://www.investopedia.com/articles/01/030701.asp
Hens, T., & Meier, A. (2016). Credit Suisse. (C. S. AG, Ed.) Recuperado el 2024, de file:///C:/Users/User/Desktop/wp-07-behavioral-finance-en.pdf
Hryshko, A., & Downs, T. (2007). System for foreign exchange trading using genetic algorithms and reinforcement learning. International Journal of Systems Science, 35(13-14), 763-774. doi:https://doi.org/10.1080/00207720412331303697
Investing. (29 de Diciembre de 2023). es.investing. Obtenido de https://es.investing.com/tools/market-hours
Jiménez-Méndez, E., & Álvarez-Lamus, G. (2024). Estrategias de trading para el bono del Tesoro estadounidense usando análisis técnico y Bloomberg Professional Services. Revista CEA, 10(22), e2634. doi:https://doi.org/10.22430/24223182.2634
Junlin, H., Baoyu, W., & Yulun. D. (2023). Brief Overview of the Development on the ForeignExchange Market in China: From 2018 to 2022. Journal of Corporate Finance Management and Banking System, 9-15. doi:https://doi.org/10.55529/jcfmbs.32.9.15
Kaufman, P. (2005). Trading System and Methods. New Jersy: WILEY.
Kavin Karthik V. (2023). Applications of Machine Learning in Predictive Analysis and Risk Management in Trading. International Journal of Innovative Research in Computer Science and Technology (IJIRCST), 11(6), 2347 - 5552. doi:https://doi.org/10.55524/ijircst.2023.11.6.4
Kumbhare, P., Kolhe, L., Dani, S., Fandade, P., & Theng, D. (2021). Algorithmic Trading using Technical Indicators. 1th International Conference on Emerging Trends in Engineering & Technology - Signal and Information Processing (ICETET - SIP) (págs. 1-6). Nagpur, India: IEEE XPLORE. doi:10.1109/ICETET-SIP58143.2023.10151614
Lee, M., Chang, J., Yeh, S., & et al. (2022). pplying attention-based BiLSTM and technical indicators in the design and performance analysis of stock trading strategies. Neural Comput & Applic, 34, 13267–13279. doi:https://doi.org/10.1007/s00521-021-06828-4
Lui, Y., & Mole, D. (1998). The use of fundamental and technical analyses by foreign exchange dealers: Hong Kong evidence. Journal of International Money and Finance, 17(3), 535-545. doi:doi:https://doi.org/10.1016/S0261-5606(98)00011-4
Mashuda, A. (2021). Euler Method on Simple Pendulum Motion to Develop Stochastic Oscillator Indicator for Analyzing the USD Non-Farm Payroll Data Volatility. Journal of Physics: Conference Series, 1805. Obtenido de https://iopscience.iop.org/article/10.1088/1742-6596/1805/1/012001
Miranda, N. E., & Murphy, D. A. (2023). ESAN. Obtenido de https://repositorio.esan.edu.pe/bitstream/handle/20.500.12640/3443/2023_MAF_20-2_01_TI.pdf?sequence=1&isAllowed=y
Mordor Intelligence Research & Advisory. (09 de 2023). Mordor Intelligence. Obtenido de https://www.mordorintelligence.com/es/industry-reports/algorithmic-trading-market
Morris, G. (2006). Candlestick charting explained: timeless techniques for trading and futures. New York: McGraw-Hill.
Munkhdalai, T., Munkhdalai, K. H., Park, H. G., Lee, M., & Li & K. H. Ryu. (2019). Mixture of Activation Functions With Extended Min-Max Normalization for Forex Market Prediction. IEEE Access, 1, 183680-183691. doi:10.1109/ACCESS.2019.2959789
Murphy, J. (2000). Análisis Técnico de losMercados Financieros. New York: New York Institute of Finance.
Nabipour, M., Nayyeri, P., Jabani, H., & Mosavi, A. (2020). Predicting Stock Market Trends Using Machine Learning and Deep Learning Algorithms Via Continuous and Binary Data; a Comparative Analysis. IEEE Access, 9, 150199-150212. doi:10.1109/ACCESS.2020.3015966
Nickolaevich, L., Igorevna, G., & Grigorievich, R. (2020). Generating a Multi-Timeframe Trading Strategy based on Three Exponential Moving Averages and a Stochastic Oscillator. International Journal of Technology, 11(6), 1233-1243. doi:https://doi.org/10.14716/ijtech.v11i6.4445
Pemy, M. (2022). Optimal Trading Algorithms under Regime Switching. The Journal of Financial Data Science, 5(4). doi:10.3905/jfds.2022.1.092
Pruitt, G. (2016). The Ultimate Algorithmic Trading System Toolbox + Website: Using Today's Technology To Help You Become A Better Trader. John Wiley & Sons.
Rosenstreich, P. (2005). Forex Revolution: An Insider’s Guide to the Real World of Foreign Exchange Trading. FT Press.
Salkar, T., Shinde, A., Tamhankar, N., & Bhagat, N. (2021). Algorithmic Trading using Technical Indicators. International Conference on Communication information and Computing Technology (ICCICT) (págs. 1-6). Mumbai, India: IEEE Xplore. doi:10.1109/ICETET-SIP58143.2023.10151614
Sarasa-Cabezuelo, A. (2023). Development of a Backtesting Web Application for the Definition of Investment Strategies. Knowledge, 3(3), 414-431. doi:https://doi.org/10.3390/knowledge3030028
Shah. (05 de 02 de 2019). Businesswire. Obtenido de https://www.businesswire.com/news/home/20190205005634/en/Global-Algorithmic-Trading-Market-to-Surpass-US-21685.53-Million-by-2026
Shanmugam, R. (2023). Algorithmic trading and quantitative strategies. Journal of Statistical Computation and Simulation, 93(9), 1478. doi:https://doi.org/10.1080/00949655.2023.2168168
Simanjuntak, T., Sadalia, I., & Irawati, N. (2021). TECHNICAL ANALYSIS AS THE BASIS FOR DECISION MAKING IN STOCK INVESTMENT IN PT. BANK MESTIKA DHARMA.TBK IN THE INDONESIAN CAPITAL MARKET (DURING THE COVID PANDEMIC JANUARY 2020 - JUNE 2021). International Journal of Economic, Business, Accounting, Agriculture Management and Sharia Administration, 3(5), 1474-1490. doi:https://doi.org/10.54443/ijebas.v3i5.1085
Taylor, M. P., & Allen, H. (1992). The use of technical analysis in the foreign exchange market. Journal of International Money and Finance, 11(3), 304-314. doi:https://doi.org/10.1016/0261-5606(92)90048-3
Titov, S. (2007). An Adaptive System of Decision Making for Financial Markets. Applied Econometrics, Russian Presidential Academy of National Economy and Public Administration (RANEPA), 7, 27-43. Obtenido de https://ideas.repec.org/a/ris/apltrx/0142.html
Wiecki, T., Campbell, A., Lent, J., & Stauth, J. (2016). All That Glitters Is Not Gold: Comparing Backtest and Out-of-Sample Performance on a Large Cohort of Trading Algorithms. The Journal of Investing, 25(3), 69. doi:10.3905/joi.2016.25.3.069
Wu, B., & Han, X. (2023). Intelligent trading strategy based on improved directional change and regime change detection. Obtenido de https://arxiv.org/pdf/2309.15383.pdf
Yafouz, A., Najah, A., Zaini, N., Sherif, M., Sefelnasr, A., & El-Shafie, A. (2021). Hybrid deep learning model for ozone concentration prediction: comprehensive evaluation and comparison with various machine and deep learning algorithms. Engineering Applications of Computational Fluid Mechanics, 15(1), 902-933. doi:https://doi.org/10.1080/19942060.2021.1926328
yahoo!finance. (31 de 12 de 2023). finanzas.yahoo. Obtenido de https://es-us.finanzas.yahoo.com/
Yazdi, S., & Lashkari, Z. (2013). Technical analysis of Forex by MACD Indicator. International Journal of Humanities and Management Sciences (IJHMS), 159-165. Obtenido de http://journalsweb.org/siteadmin/upload/P413021.pdf
Yensen, N., Yi-Ching, L., & Paoyu, H. (2015). Momentum in the Chinese Stock Market: Evidence from Stochastic Oscillator Indicators, Emerging Markets Finance and Trade. Emerging Markets Finance and Trade, 15, S99-S110. doi:https://doi.org/10.1080/1540496X.2014.998916
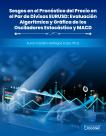
Descargas
Publicado
Colección
Licencia

Esta obra está bajo una licencia internacional Creative Commons Atribución-NoComercial-SinDerivadas 4.0.